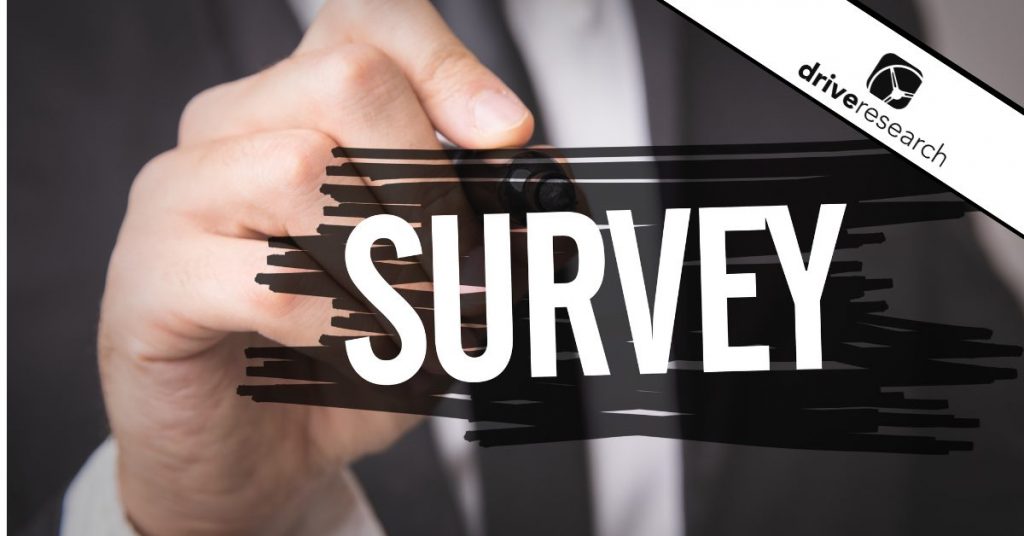
You can always learn something interesting when you include an open-ended question in an online survey. They are most beneficial when there is any uncertainty about the answers a respondent might provide to your question. With an open-ended question, researches can give respondents a text box and let them speak their mind.
It’s always great to use this method of gathering data, but how can you efficiently sort all of the replies if you have 1000s of respondents?
The truth is, there is no one perfect way to turn all those open-ended responses into clear-cut findings. Each approach has its own pros and cons and may just depend on the situation.
That’s why we’re showing the best approaches our survey company find most helpful when reviewing open-ended responses!
There are several enticing choices when it comes to analyzing open-ended responses from an online survey. Check out the most useful approaches below.
#1. Code Responses into Buckets
A common use of open-ended text data is coding. Through this approach, individual responses are assigned a numerical code. Each code represents a bucket or segment consisting of similar responses. For example, I may code the response, “The software was easy to use.” under the bucket “Ease of Use.”
Once each response has been assigned a code, the text data can then be quantified and treated like variables in a “Select One” or “Select All That Apply” question format. A market research company can also run an analysis on this numeric data to create cross-tabulations and charts.
The downside with coding is that it can be a manual and time-consuming process. Traditionally, a researcher will sift through the open-ended responses one by one and decide what code or codes are the best fit. Though tedious, this is a very accurate way to bucket responses into larger themes.
#2. Share the Full List
Another valid way to present the open-ended responses in a survey is to simply provide the entire list of text. The best practice here includes cleaning the responses to make them client-ready. This process consists of proofing for spelling, grammar, punctuation, proper nouns, and inappropriate responses.
The key benefit with this approach is the ability for a client to read through all the answers in their original detail. Sharing the full list also makes sense when many of the responses contain a few sentences or more. Understanding the context of these responses will help get the most out of the data.
Drawbacks of sharing the entire set of open-ended responses are centered around the time it takes to clean them and any consequences of sharing unfiltered responses with the client. Consider how long it may take to review and correct every single response before promising to deliver the open-ended responses. Some unforgiving feedback in the responses may also do more harm than good.
Here are 4 tips for quality checking survey data, including cleaning your open-ends.
#3. Input the Text into a Word Cloud
Word clouds offer a fast, low-effort way to identify the buzz words across all open-ended responses. Typically constructed with a software, word clouds are visualizations based on the frequency of every mentioned word. Phrases can also be kept together, if needed.
The size of a word within the cloud immediately tells the reader what words are important to the question that was asked. This approach works especially well when the associated question only asks for a word or phrase.
Word clouds are less helpful in situations where the responses are multiple sentences or otherwise complicated in nature. There is often little context when reading a word cloud, as well. You aren’t getting the full story, just the key words.
#4. Use Text Analytics
Automation is increasingly being applied to open-ended responses from surveys. These new tools attempt to make it as efficient as possible to get answers from the text data with minimal human intervention.
One of these techniques is automated coding, in which artificial intelligence is used to code open-ended responses into buckets automatically. This is usually a huge time-saver, but the accuracy of coding is still not on par with manual coding.
Another example of text analytics is sentiment analysis. Existing tools can determine the sentiment of an individual response to see if it is negative, neutral, or positive. This technique is most useful for unstructured text data like social media posts.
#4. #5 Use a Spreadsheet
Spreadsheets will help you analyze the data visually in a .csv or excel file. This is a great option especially if you’re a pro at excel. There are a ton of things you can automatically do inside excel to sort data. Once you’ve imported the data, you’re able to sort everything from individual answers even to give you a high-level overview of the data.
Even from excel you can create multiple different types of graphs, charts, and more from the spreadsheet. That way it gives you even more ways on top of the spreadsheet to view the data.
Contact Drive Research
Drive Research is a market research company. Our team works with open-ended responses on a daily basis and can recommend the best approach for you.
Interested in learning more about our market research services? Reach out today.